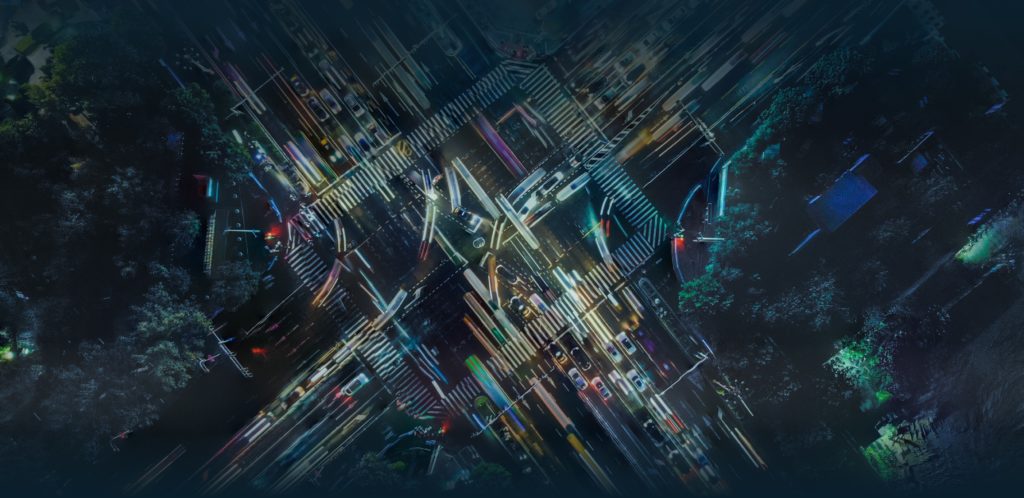
Researchers Zuoxian Gan, Min Yang, Tao Feng and Harry Timmermans published an article on Springer Nature on 2018, regarding the urban mobility patterns.The article provides a up-to-date review on this issue. Here there are some of the key issues.
Smart card data derived from automatic fare collection (AFC) systems of public transit enable us to study resident movement from a macro perspective. The rhythms of traffic generated by different land users differ, reflecting differences in human activity patterns. Thus, an understanding of daily ridership and mobility patterns requires an understanding of the relationship between daily ridership patterns and characteristics of stations and their direct environment.
Unfortunately, few studies have investigated this relationship. Researchers stress that this study aims to propose a framework of identifying urban mobility patterns and urban dynamics from a spatiotemporal perspective and pointing out the linkages between mobility and land cover/land use (LCLU). Relying on 1 month’s transactions data from the AFC system of Nanjing metro, the 110 metro stations are classified into 7 clusters named as employment-oriented stations, residential-oriented stations, spatial mismatched stations, etc., each characterized by a distinct ridership pattern (combining boarding and alighting).
The analytical framework and findings may be beneficial for improving service efficiency of public transportation and urban planning. The authors consider that this study presents one of the first attempts of exploring the relationship between local LCLU and metro ridership patterns, while at the same time analyzing the layout of urban functions in a Chinese city.
The emergence of information and communication technologies (ICT) and the rise of big data have advanced research on travel behavior, activity mining, mobility flows and mobility regularities Big data with specific time–space information of travelers make it possible to analyze urban mobility from a spatiotemporal perspective. Moreover, the sample size of these datasets also improves the accuracy of the analysis. Big travel data can be divided into GPS devices, taxi trajectories, mobile phone data, smart card data (SCD) and social media data.
In contrast to taxi trajectories and social medial check-in data, the other three types of data have two salient advantages: high spatiotemporal precision and tracking long-term resident movements.
Study area and data collection
Nanjing, the heart of Nanjing Metropolitan, is the capital of Jiangsu Province and one of most important political, economic, and cultural centers in eastern China, known also by its famous historical and cultural richness. It covers a total of 6587.02 square kilometers.
The city represents the typical microcosm of Chinese cities, which are still under rapid population urbanization and urban growth. Its total population increased from 4.7 million in 1985 to 8.27 million in 2016 and the number of urban residents increased to 6.78 million, accounting for 82% increase. For the purpose of analysis, the dataset was first aggregated and converted into hourly records.
There were 21 weekdays, 8 weekends and 1 holiday in April 2015. Researchers focused on people’s travel patterns on weekday and the records of 9 non-weekdays were not considered.
In addition to the metro trip dataset, the researchers also used the land use data of the Nanjing region from the Nanjing Urban Planning Bureau (NUPB).
Discussion and conclusions
As the authors of the article explain, the results show that, among these stations, the employment-oriented stations and the mixed mainly employment-oriented stations have the highest ratios of afternoon peak hourly boarding and morning peak hourly alighting. Meanwhile, the residential-oriented stations and the mixed mainly residential- oriented stations have the highest ratios of afternoon peak hourly alighting and morning peak hourly boarding in weekdays.
The results of the multinomial logit model confirm that the spatial distribution of different types of metro stations reflects the spatial distribution of different urban functions. The researchers highlight that the results of the study may have some implications for Nanjing urban design and transit planning.
The implication of this finding may be important for transit operators to work out suitable train schedules to improve service efficiency. Moreover, these results may especially be useful for daily arrangements and services in metro stations, especially the connection between metro and other transportation modes. In terms of medium- and long-term planning, these results can help planners better understanding urban mobility.
The authors of the article acknowledge that the metro system is a restricted representation of urban mobility although it carries a large volume of passengers in a city and plays an important role in daily travel. The approach needs to be extended certainly to other traffic modes such as bus, tram etc. to enrich the analysis.
The integration of more transportation modes may be considered more accurate to understand urban mobility patterns and the link between mobility patterns and urban land use. Lastly, the conceptual framework proposed in the present paper may be applied in other cities having rail transit systems. Comparative and empirical studies across cities are encouraged in the future.